Research Projects
Imagine you’re driving in a conditionally automated vehicle with an in-vehicle intelligent agent, and it suddenly asks you to take over. The agent is able to provide information about what it's doing, why it's doing it, and what it plans to do next that can help you to understand system's intention and make better performance. But how much information do you really need from the system? Does more information always lead to better performance? Could too much information overwhelm you instead?​
​
To explore these questions, we conducted an experiment using a driving simulator. We used two robots to represent different systems with varying reliability to test how the amount of information provided affects both human perception and performance.
No driving system is perfect. While having an in-vehicle intelligent agent (IVIA) as an assistant is generally better than having none, if the agent isn’t reliable, will people still trust and use it? Would you want to ask for more information if you are confused? Will the availability for the agent to "communicate" gives you a sense of more human-like and trigger more trust behavior and perception?
​​
To explore these questions, we tested two types of IVIAs: a proactive (Push) system that provides all relevant information about an event at once, and an on-demand (Pull) system that only gives information when the driver requests it. Additionally, we conducted a survey to understand users' preferences for these interactions and explored the reasons behind their choices.
What is the best way for communication and collaboration?
As automated vehicles (AVs) shift the driver’s role from active controller to passive observer, establishing trust becomes increasingly critical. Given the fundamental advancements in vehicle capabilities, it is important to consider the driver-vehicle relationship as a team.
Our on-going research considers adding social elements into the voice agents in enhancing human-AI teaming, exploring the design implications considering the system's capability and human capability, identifying common obstacles in designing driver-ADS teams, and suggesting potential solutions. We hope to offer valuable insights for designing AI teammates that are perceived as trustworthy, relatable, and capable partners within human-AI teams (HATs).
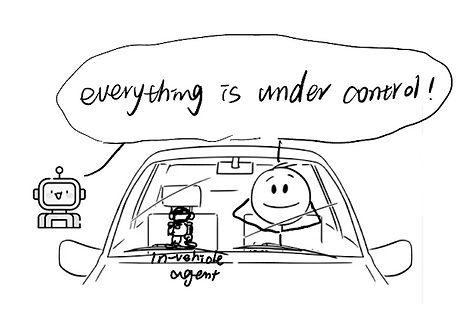
Other Interesting Research Projects
I am Involved With

To address the challenge of real-time assessment of human trust, we designed a simulated driving study. By implementing machine learning classification models, we are able to identify the most efficient combination of different psychophysiological sensors (i.e. Eye-tracking, ECG, EEG, EDA) and visualize and identify the features that contribute the most to predictive outcomes.

Challenges Faced by Older Adults in Automated Vehicle Adoption
Older adults are poised to be one of the groups that benefit the most from the widespread adoption of autonomous vehicles (AVs). However, numerous studies have found that they face significant challenges in adopting this technology. To address this, we conducted a literature review summarizing research from the past decade, focusing on the barriers and difficulties older adults encounter with AV adoption. This review highlights key factors influencing their acceptance and provides insights into how future developments can be tailored to meet their needs. We presented a lecture on the 68th HFES annual meeting (ASPIRE) to deliver our results.
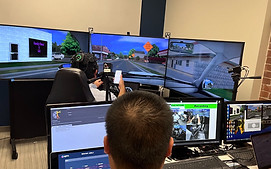
Automated Flagger Systems
Flaggers work closely to open traffic lanes which makes them a high-risk profession. To eliminate such hazardous tasks, we are testing a range of current Automated Flagger Assistance Devices (AFAD) systems to evaluate their effectiveness in various environments. These systems aim to improve safety by reducing the need for human involvement in dangerous flagging operations while maintaining efficient traffic control in construction zones. This project is funded by the Indiana Department of Transportation.
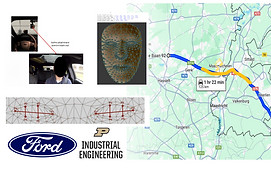
Anti-drowsy-driving Methods
Drowsy driving is one of the most critical factors that cause death on the road. To solve the problem, we conducted both naturalistic driving and simulated study to investigate several invigorate methods for keeping awakeness and mitigating the driver's drowsy level. This project is a joint collaboration with Ford Motor Company.

Design a Home Device With LLM for Older Adults
As people age, they face certain challenges in their daily affairs, particularly considering memory decline. A home device can assist in assessing how older adults interact with digital assistants using both voice and visual inputs. In our usability testing, we developed user personas and designed a series of methods to measure the effectiveness of this future technology. We assessed task completion rates, the number of times users had to repeat themselves, and their ability to interact with the device under conditions of voice or visual impairment.
This project report earned first place at the Student Career and Professional Day during the 68th HFES annual meeting (ASPIRE).

Human Factors in
Nuclear Power Plant
Eliminating human error during the commissioning phase is critical for ensuring safety and system reliability. For this project, I was responsible for analyzing and improving system reliability by addressing human errors and technical issues. I retrieved and reviewed over 90 incident reports, conducted thorough root cause analysis using data visualization techniques, and proposed effective mitigation strategies to enhance system reliability and minimize the occurrence of human errors. This work was summarized in my Bachelor's thesis, which won the Best Thesis Award in 2019.